|
ACCESS THE FULL ARTICLE
No SPIE Account? Create one
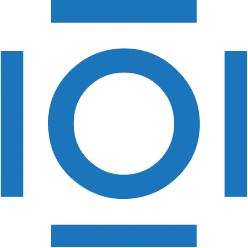
CITATIONS
Cited by 1 scholarly publication and 3 patents.
Optical proximity correction
Neural networks
Machine learning
Photomasks
Fourier transforms
Lithography
Neurons