|
ACCESS THE FULL ARTICLE
No SPIE Account? Create one
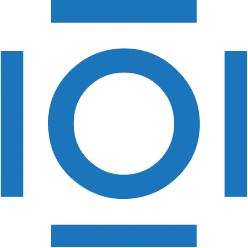
CITATIONS
Cited by 3 scholarly publications.
Electromyography
Neural networks
Gesture recognition
Feature extraction
Motion models
Sensors
Artificial neural networks