|
ACCESS THE FULL ARTICLE
No SPIE Account? Create one
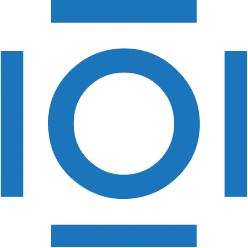
CITATIONS
Cited by 1 scholarly publication.
Reflectivity
Monte Carlo methods
X-rays
Structural design
Machine learning
Materials science