|
ACCESS THE FULL ARTICLE
No SPIE Account? Create one
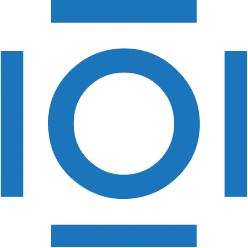
CITATIONS
Cited by 2 scholarly publications.
Image segmentation
Network architectures
Cardiovascular magnetic resonance imaging
Magnetic resonance imaging
Medical imaging
Convolutional neural networks