|
ACCESS THE FULL ARTICLE
No SPIE Account? Create one
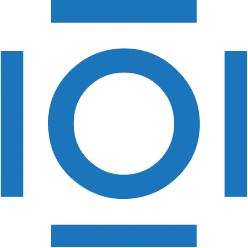
CITATIONS
Cited by 1 scholarly publication.
Blood
Image segmentation
Algorithms
Bone
Image processing algorithms and systems
Solids
Binary data