|
ACCESS THE FULL ARTICLE
No SPIE Account? Create one
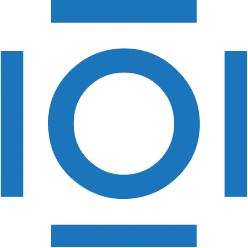
CITATIONS
Cited by 5 scholarly publications.
Turbulence
Fourier transforms
Spatial frequencies
Atmospheric modeling
Atmospheric propagation
Monte Carlo methods
Statistical modeling