|
ACCESS THE FULL ARTICLE
No SPIE Account? Create one
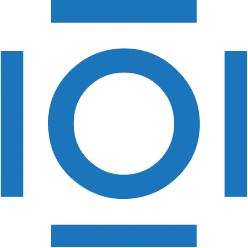
CITATIONS
Cited by 2 scholarly publications.
Independent component analysis
Functional magnetic resonance imaging
Data modeling
Neural networks
Solids
Data acquisition
Signal to noise ratio