|
ACCESS THE FULL ARTICLE
No SPIE Account? Create one
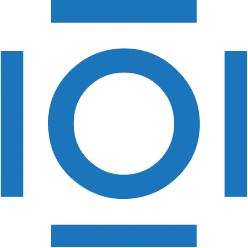
CITATIONS
Cited by 2 scholarly publications.
Missiles
Particle filters
Particles
Sensors
Filtering (signal processing)
Optical filters
Nonlinear filtering