|
ACCESS THE FULL ARTICLE
No SPIE Account? Create one
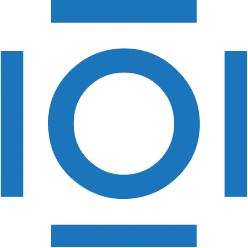
CITATIONS
Cited by 35 scholarly publications.
Detection and tracking algorithms
Sensors
Algorithm development
Mining
Data modeling
Performance modeling
Feature extraction