|
ACCESS THE FULL ARTICLE
No SPIE Account? Create one
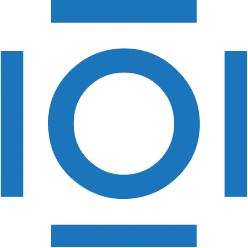
CITATIONS
Cited by 9 scholarly publications.
Matrices
Compressed sensing
Atrial fibrillation
Optical spheres
Probability theory
Space operations
Computational mathematics