|
ACCESS THE FULL ARTICLE
No SPIE Account? Create one
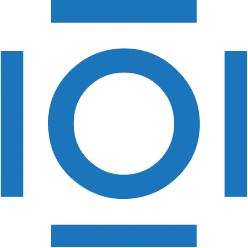
CITATIONS
Cited by 3 scholarly publications.
Atrial fibrillation
Machine learning
Modulators
Image classification
Statistical analysis
Image segmentation
Luminescence