|
ACCESS THE FULL ARTICLE
No SPIE Account? Create one
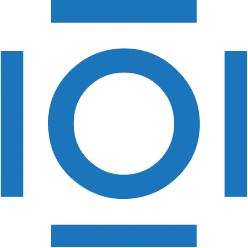
CITATIONS
Cited by 1 scholarly publication.
Sensors
Spatial resolution
Hyperspectral imaging
Sensor fusion
Image fusion
RGB color model
Signal to noise ratio