|
ACCESS THE FULL ARTICLE
No SPIE Account? Create one
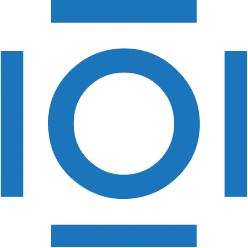
CITATIONS
Cited by 2 scholarly publications.
Algorithms
Error analysis
Information technology
Linear filtering
Matrices
Principal component analysis
Data processing