|
ACCESS THE FULL ARTICLE
No SPIE Account? Create one
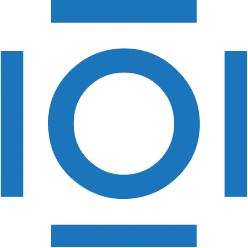
CITATIONS
Cited by 1 scholarly publication.
Expectation maximization algorithms
Feature selection
Computer networks
Data modeling
Sensors
Autoregressive models
Data transmission