|
ACCESS THE FULL ARTICLE
No SPIE Account? Create one
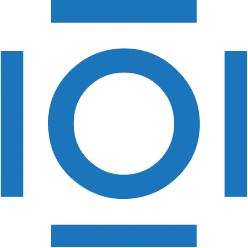
CITATIONS
Cited by 17 scholarly publications.
Earth observing sensors
Landsat
Image processing
Mendelevium
Error analysis
Reflectivity
Mining