|
ACCESS THE FULL ARTICLE
No SPIE Account? Create one
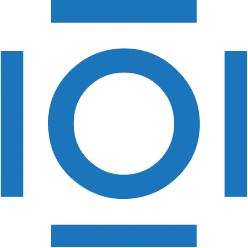
CITATIONS
Cited by 20 scholarly publications.
Vegetation
Target detection
Hyperspectral imaging
Calibration
Hyperspectral target detection
Reflectivity
Sensors