|
ACCESS THE FULL ARTICLE
No SPIE Account? Create one
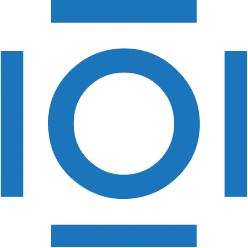
CITATIONS
Cited by 28 scholarly publications.
Synthetic aperture radar
Convolution
Convolutional neural networks
Image classification
Stanford Linear Collider
Lithium
Data modeling