Accurately mapping tea plantation distribution is crucial to environmental protection and sustainable development. Hyperspectral and synthetic aperture radar (SAR) data have recently been widely used in land cover classification, but their ability to extract tea plantation regions still needs to be confirmed. Compared with traditional pixel-based image analysis (PBIA), object-based image analysis (OBIA) for tea plantation mapping is more worthy of implementation. This study explored the performance of Gaofen-5 (GF-5) and copolarized SAR data for tea plantation mapping using pixel- and object-based support vector machine algorithms in Wuyishan, China. Comparison of PBIA and OBIA demonstrated the significant differences in visual effect and classification accuracy. The object-based classifications especially offered a more contiguous depiction with fewer speckles of tea plantations than pixel-based classifications did. Moreover, object-based classifications improved overall accuracy (OA) between 1.7% and 7.9% in all scenarios when compared to pixel-based classifications. As for datasets, classifications using only GF-5 data obtained an OA of over 85%, while fusing images decreased classification accuracy due to the lower separability between tea and forest, showcasing that the fusion of hyperspectral and SAR data does not guarantee the improvement of classification accuracy. The integration of GF-5, horizontal transmit and horizontal receive (HH), and vertical transmit and vertical receive (VV) polarized data outperformed other data combinations in both pixel- and object-based classifications and achieved the highest OA (95.58%) in OBIA, with a 98.13% producer accuracy and 93.34% user accuracy of tea plantations. The results indicated OBIA could overcome the shortcomings of the PBIA and effectively improve the mapping accuracy of tea plantation, and OBIA integrating GF-5, HH, and VV polarized data could play a distinguished role in tea plantation mapping. This work provides a promising approach for mapping tea plantations and demonstrates that the integration of GF-5 and copolarized data can improve spectral separability of vegetation, which is also significant for general forest mappings. |
ACCESS THE FULL ARTICLE
No SPIE Account? Create one
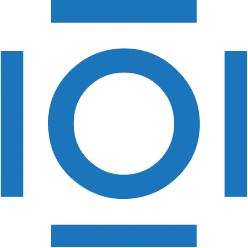
CITATIONS
Cited by 2 scholarly publications.
Synthetic aperture radar
Associative arrays
Image analysis
Image classification
Image segmentation
Image fusion
Vegetation