In the tracking-by-detection scheme of multiple object tracking (MOT), the data association process in which existing tracking data and new detections are matched over time is very important. A framework is proposed to solve the data association problem in MOT in scenarios where there are potential target interactions and occlusions in crowded environments. This framework consists of an input layer and an association layer. The input layer is an end-to-end feature-map extraction model that incorporates a simplified Siamese convolutional neural network, which effectively distinguishes similar objects based on their appearance and motion. The association layer is composed of a bidirectional gated recurrent unit network with three layers of fully connected networks (FCNs), whose outputs are fed into the FCNs and transformed into an association matrix that reflects the matching scores between the detections and existing tracks. The matrix is then used to minimize the loss of the framework. The experimental results show that the proposed framework demonstrates outstanding performance for MOT, with its accuracy and precision in MOT reaching values as high as 26.1% and 71.2%, respectively. |
ACCESS THE FULL ARTICLE
No SPIE Account? Create one
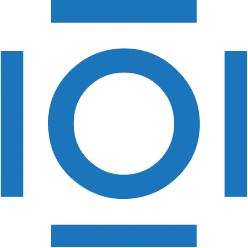
CITATIONS
Cited by 1 scholarly publication.
Video
Data processing
Target detection
Convolutional neural networks
Data modeling
Video surveillance
Machine vision