In recent years, to save bandwidth and storage space, images are usually compressed to reduce data volume, which leads to the loss of image details and affects the super-resolution (SR) performance. SR of compressed images is a key technique for addressing this problem. We propose the compressed-image super-resolution using enhanced attention network (CISREAN). First, the task is divided into two subtasks: image decompression and SR. Each subprocess introduces an enhanced residual module (ERM) with an attention mechanism. The ERM consists of several wide-activation residual blocks (WARBs) and an attention unit called the cascading residual attention (CRA) block. A WARB achieves better reconstruction than traditional image processing with the same computational complexity, and the CRA extracts more useful information in feature mapping even with fewer channels. This makes the ERM light and effective. Next, initial features are selected for wider inception and less blocking by overlapping extractions from the compressed image during decompression by convolutional layers. After completing both subprocesses, an end-to-end network is trained; it reduces compression artifacts, performing SR simultaneously. Extensive experiments on JPEG images with different quality factors show that CISREAN provides state-of-the-art performance based on objective metrics and subjective visual quality. |
ACCESS THE FULL ARTICLE
No SPIE Account? Create one
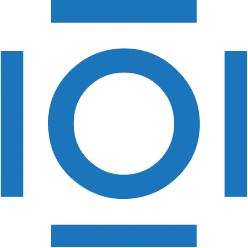
CITATIONS
Cited by 1 scholarly publication.
Image compression
Super resolution
Lawrencium
Image processing
Convolution
Feature extraction
Reconstruction algorithms