Purpose: Recent research has been producing an important effort in encoding of digital image content. Most of the adopted paradigms only focus on local features and lack of information about location and relationships between them. Approach: To fill this gap, we propose a framework built on three cornerstones. First, the adoption of attributed relational scale-invariant feature transform regions graph, for image representation. Second, the application of a graph embedding model, to work in a simplified vector space, is performed. Finally, fast graph convolutional networks address classification task on a graph-based dataset representation. Results: The framework is evaluated on state of art object recognition datasets with uniform background. Conclusions: A wide experimental phase is performed through a comparison to well-known competitors. |
ACCESS THE FULL ARTICLE
No SPIE Account? Create one
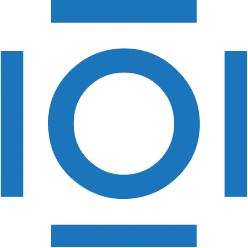
CITATIONS
Cited by 5 scholarly publications.
Object recognition
Vector spaces
Feature extraction
Mining
Image processing
Prototyping
Data modeling