Video action recognition methods based on deep learning can be divided into two types: two-dimensional convolutional networks (2D-ConvNets) relied and three-dimensional convolutional networks (3D-ConvNets) relied. 2D-ConvNets are more efficient to learn spatial features, but cannot capture temporal relationships directly. 3D-ConvNets can jointly learn spatial–temporal features, but their learning is time-consuming because of a large number of networks’ parameters. We therefore propose an effective spatial–temporal interaction (STI) module. The 2D spatial convolution and the one-dimensional temporal convolution are combined through attention mechanism in STI to learn the spatial–temporal information effectively and efficiently. The computation cost of the proposed method is far less than 3D convolution. The proposed STI module can be combined with 2D-ConvNets to obtain the effect of 3D-ConvNets with far fewer parameters, and it can also be inserted into 3D-ConvNets to improve their ability to learn spatial–temporal features, so as to improve the recognition accuracy. Experimental results show that the proposed method outperforms the existing counterparts on benchmark datasets. |
ACCESS THE FULL ARTICLE
No SPIE Account? Create one
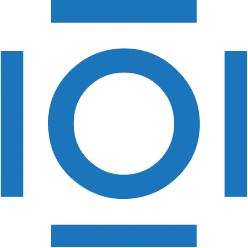
CITATIONS
Cited by 1 scholarly publication.
Video
Convolution
Network architectures
RGB color model
Optical flow
3D modeling
Feature extraction