|
ACCESS THE FULL ARTICLE
No SPIE Account? Create one
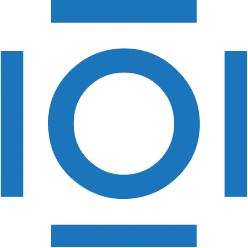
CITATIONS
Cited by 24 scholarly publications.
Tissues
3D modeling
Magnetic resonance imaging
Brain
Ischemic stroke
Performance modeling
Data modeling