Defects in semiconductor processes can limit yield, increase overall production cost, and also lead to time-dependent critical component failures. Current state-of-the-art optical and electron beam (EB) inspection systems rely on rule-based techniques for defect detection and classification, which are usually rigid in their comparative processes. This rigidity limits overall capability and increases relative engineering time to classify nuisance defects. This is further challenged due to shrinkage of pattern dimensions for advanced nodes. We propose a deep learning-based workflow that circumvents these challenges and enables accurate defect detection, classification, and localization in a unified framework. In particular, we train convolutional neural network-based models using high-resolution EB images of wafers patterned with various types of intentional defects and achieve robust defect detection and classification performance. Furthermore, we generate class activation maps to demonstrate defect localization capability of the model “without” explicitly training it with defect location information. To understand the underlying decision-making process of these deep models, we analyze the learned filters in pixel space and Fourier space and interpret the various operations at different layers. We achieve high sensitivity (97%) and specificity (100%) along with rapid and accurate defect localization. We also test performance of the proposed workflow on images from two distinct patterns and find that in order to retain high accuracy a modest level of retraining is necessary. |
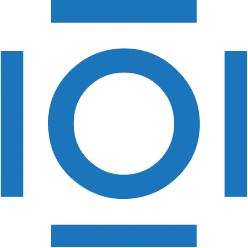
CITATIONS
Cited by 9 scholarly publications.
Data modeling
Convolution
Semiconductors
Defect detection
Performance modeling
Image classification
Semiconducting wafers