|
ACCESS THE FULL ARTICLE
No SPIE Account? Create one
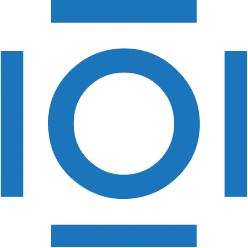
CITATIONS
Cited by 24 scholarly publications.
Principal component analysis
Image filtering
Neural networks
Feature extraction
Data modeling
Image processing
Detection and tracking algorithms