|
ACCESS THE FULL ARTICLE
No SPIE Account? Create one
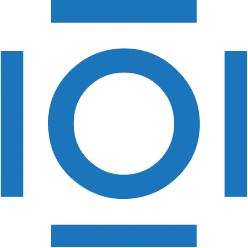
CITATIONS
Cited by 9 scholarly publications.
Source mask optimization
Photomasks
Lithography
Standards development
Algorithm development
Diffraction
Resolution enhancement technologies