|
ACCESS THE FULL ARTICLE
No SPIE Account? Create one
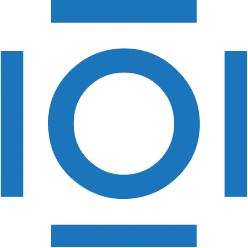
CITATIONS
Cited by 13 scholarly publications and 1 patent.
Lung cancer
Cancer
Lung
Diagnostics
Network architectures
Neural networks
Medical imaging